Large language models (LLMs) like Chat-GPT and Google’s Bard are rapidly changing the way we interact with datasets that are simply too big, complex, and varied for any ordinary person to comprehend.
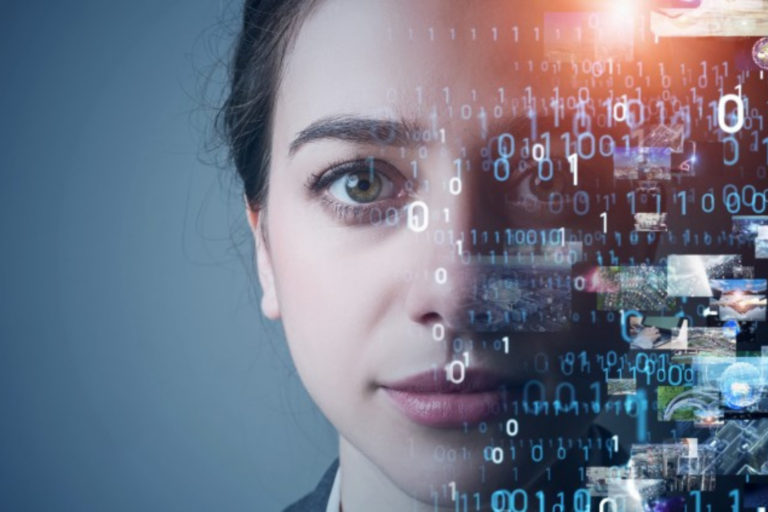
These artificial neural networks take millions or billions of parameters into account as they train on enormous quantities of data, allowing them to do everything from accurately summarize text and create unique artwork to closely mimic authentic human conversations.
The meteoric rise of LLMs has sparked intense interest in the healthcare industry: how can these models be used to make discoveries easier, improve outcomes, lower costs and produce better experiences for patients and staff alike?
There are nearly endless opportunities to use artificial intelligence to answer these questions, and plenty of projects are already underway to apply LLMs to some of the most pressing problems in clinical care and life sciences.
But how do LLMs fit into the oncology space, where the data is more-than-usually dense and less-than-typically accessible? Are we ready to take advantage of everything LLMs have to offer, or do we still have more work to do before these models can truly shine? What factors do we need to consider as we explore the potential for LLMs to support continued innovation in cancer care?
LLMs need data in order to perform desired tasks reliably and accurately, and they need lots of it. For example, the Davinci generative pre-trained transformer (GPT) model from OpenAI and Microsoft uses 175 billion parameters and 45 terabytes of text data to support its natural language processing capabilities.
The data required to train these models also needs to be of high quality, especially if used for healthcare applications. Missing or poorly standardized data elements can affect the way the model learns and produces results.
Unfortunately, oncology data is still very siloed and poorly standardized, which makes it difficult to access and aggregate for training. And since “cancer” is really just a broad label for hundreds of different diseases with dozens of subtypes, finding enough training data on each type of cancer to support a robust LLM is challenging.
As we work to create and collect enough high-quality, community-wide data to feed larger and larger models over time, LLMs will become increasingly viable in oncology and other specialties where the quality and quantity of the data both matter to the ultimate outcome.
LLMs can perform many different tasks, from answering questions to generating entirely new content. To train a model correctly, we need to start by understanding exactly what we want out of it. Is it designed to provide clinical decision support? To aid with clinical documentation? To discover new molecules to pursue for drug development? Or to predict outcomes for patients?
LLMS are already being infused into the front end of the clinical care cycle, with major electronic health IT firms like Nuance, Epic Systems, and eClinicalWorks recently announcing LLM integrations to smooth out workflows, foster patient engagement, and reduce user burdens.
However, taking LLMs one step further into the realm of research and discovery will demand more intricate models that have oncology-specific data and training layered on top of the core of natural language processing that enables Chat-GPT and its competitors to exhibit logical “thought” and produce easily consumable results.
It’s important for oncology decision-makers to understand that they may need to create bespoke models, or modify off-the-shelf options, before they can be used for these more advanced objectives, such as identifying the most successful treatments for a particular disease, uncovering disparities in outcomes among certain groups, or observing the use of a test or drug over time.
Clearly identifying the desired use case before beginning work on an LLM will be crucial for maximizing investment and ensuring that the model will meet expectations once it’s applied in the field.
Bias is a perennial concern in all data analytics, but especially in LLMs and other advanced AI models that continuously learn from their past experiences. Bias that creeps in at the beginning of the training process will be magnified exponentially over time if not addressed, making it critically important to start with the best possible data and training parameters.
Reducing bias in healthcare data means collecting information from diverse and representative patient groups, including those of varying racial, ethnic, and socioeconomic backgrounds. However, much of the existing corpus of available clinical and research data is heavily skewed toward white, male, and more affluent populations, leaving us at a disadvantage as we begin to feed the latest generation of LLMs.
Before investing too much in artificial intelligence tools, we must take steps to correct our data biases and ensure that we are starting on a firm foundation of inclusivity and representation. That means actively recruiting underrepresented communities to participate in clinical trials, funding research projects that can close knowledge gaps in health disparities, and ensuring access to top-quality cancer care for people of all backgrounds and circumstances.
Developing a more representative data pool to funnel into LLMs will ensure that we reduce bias as much as possible while increasing the availability of high-quality data, uncovering new use cases, and maximizing our opportunities to take advantage of what the next generation of artificial intelligence tools can do.