Throughout our blog series, we have talked extensively about the benefits of using real-world data (RWD) to enhance clinical trials and accelerate research into new therapies for a variety of different conditions.
Now it’s time to take a closer look at the details: what comprises real-world data? What sources of information are most valuable to collect and curate? And how can these novel data sets support the development of safe, effective therapies?
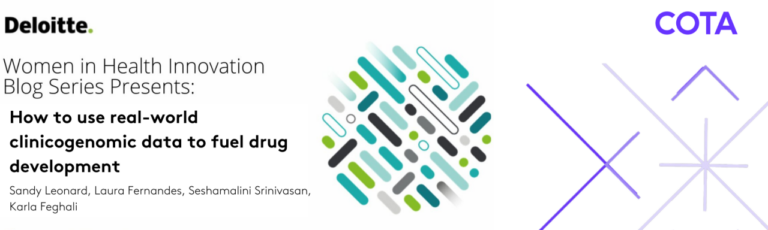
What is multimodal real-world data?
Multimodal data, or multisource data, is data that has its origins in different areas of the healthcare continuum. These data sources may include EHR data, claims, laboratory and pharmacy records, molecular profiles, medical device data, and patient-reported data on surveys, questionnaires, or even social media.
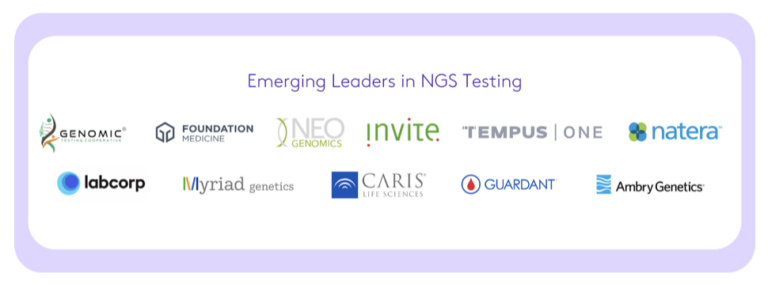
Bringing all of this data together into a curated, fit-for-purpose dataset to support research and development can be a challenge, because these data sources weren’t originally designed to fit together neatly. Instead, researchers and analysts must consider a number of different issues when synthesizing multimodal data, including how to accurately identify a single patient via tokenization or other means.
Data scientists must carefully balance the volume of data (a higher number of data sources could create a richer, more complete portrait of an individual) with the data integrity and governance issues of merging many sources together.
The challenge becomes even more complicated as we start to explore other datasets to augment our current core sources of RWD data. For example, we are increasingly integrating imaging data, personal device data, social determinants of health (SDOH) data, and patient experience data into the RWD ecosystem. These sources tend to be even “messier” than other data types due to poor standardization and high amounts of unstructured information.
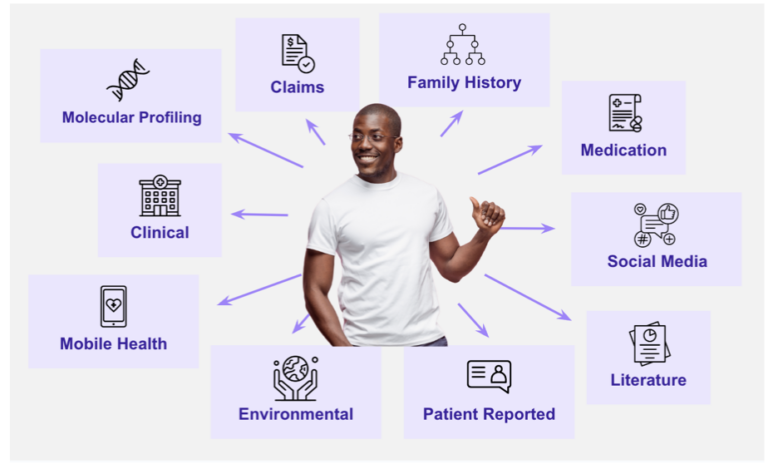
Then we get to the most complex dataset of them all: multi-omics data. As genetic testing becomes more common and our understanding of biomarkers and genetic mutations increases exponentially, researchers are eager to fully leverage genomic sequencing information as part of the clinical trial and drug development pipelines. That’s where it starts to get really interesting.
What is the value of clinicogenomics data in a multimodal RWD environment?
The cost of drug development is staggering. It can take more than $2 billion to bring a single therapy from conception to market. And since only 5% to 10% of candidate molecules ever complete the full journey, there are enormous opportunities to save costs by using better data at the beginning to guide investments and resource utilization. Since drug targets with human genetic evidence of disease association are significantly more likely than others to make it to the finish line, it’s crucial to bring genomics into the process as swiftly as possible.
The value proposition increases even further if we can appropriately integrate genomic data with other deep, rich, highly curated multimodal RWD data to create highly detailed clinicogenomics datasets and start conducting population-wide genome association studies.
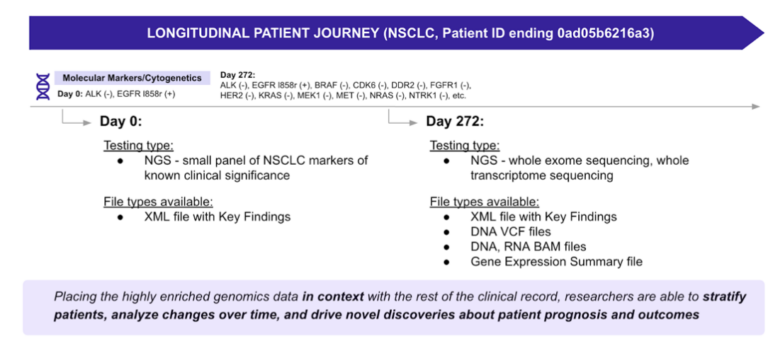
Researchers and drug developers can find the necessary genomic data in several different places, including large biobanking projects (such as All of Us, UK BioBank, and FinnGen), or specialty RWD vendors that provide data for specific applications. In either case, developers must be aware that there is substantial heterogeneity in how RWD are linked within biobanks, and understanding how this variation affects genetic findings is critical.
How can clinicogenomic data be used across the drug development lifecycle?
Clinicogenomic data can be used for a wide array of applications across the entire drug development process.
In the research phase, multimodal RWD with a genomics component can be used for target discovery and validation, characterizing disease baselines, understanding drug mechanisms of action, or drug repositioning and repurposing.
In the preclinical phase, clinicogenomics can help to identify correlations between compounds and drug response or conducting toxicology profiling to understand the potential negative effects of a drug before it’s given to human participants.
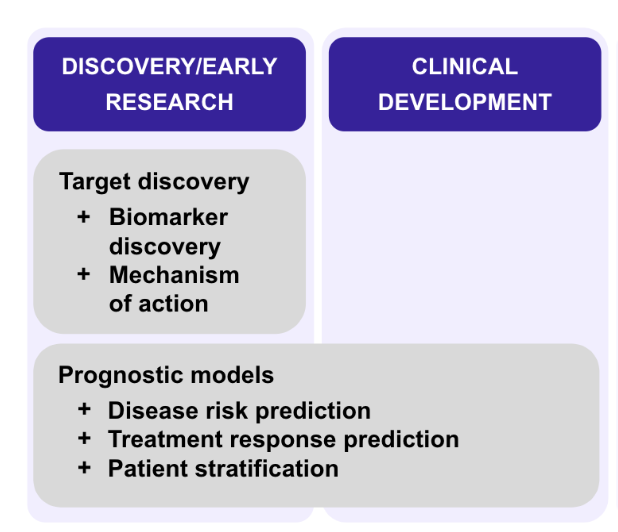
During a clinical trial, these types of datasets could help to stratify patients to increase the probability of trial success, predict outcomes or adverse events for certain populations based on their genetic factors, or even support the development of liquid biopsies using ctDNA.
There are also applications in the commercialization process. Multisource clinicogenomics data can assist with monitoring the long-term effects of a therapy as part of Phase 4 safety studies, identify any long-term secondary impacts of the product, and help to appropriately position the product in the market to ensure maximum benefit to both the developer and to patients.
These approaches are already producing benefits for patients. For example, many people cannot tolerate statin therapy for high cholesterol, despite the fact that statins are the gold standard treatment for this condition.
When researchers examined genetic data to gain understanding of the mechanism of action of high cholesterol, they found that certain mutations (proprotein convertase subtilisin kexin type 9) played a role in development of the disease. They used these insights to develop an alternate therapy using monoclonal antibodies that target the mutation. This led to the launch of two groundbreaking drugs, evolocumab, and alirocumab, that provide suitable alternatives to statin therapy.
This is a classic example of genetic evidence playing a crucial role in drug approval. With the rich clinicogenomic RWD that is available today, we are likely to see more success stories like this one alongside reduced costs and faster development of life-saving drugs for patients.