Throughout this blog series, we have taken a deep dive into the world of real world data (RWD): what it is, why it’s valuable, and how to start harnessing its potential to transform the drug development lifecycle.
Now, let’s look at the future of RWD in action and explore the world of external control arms (ECAs).
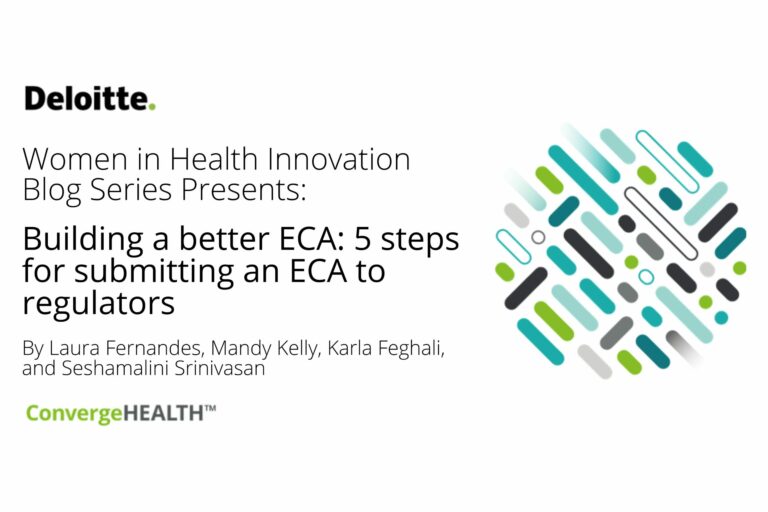
An ECA uses real-world data, completed clinical trial data, and/or other patient-level information to create an asynchronous cohort of patients who closely match the characteristics of the population in the investigational arm. ECAs allow researchers to conduct comparative analyses to the patients enrolled on the investigational single arm in situations where it is unfeasible to conduct a randomized controlled trial (RCT) due to low patient volumes, hesitancy to be randomized to the control arm or lack of an acceptable standard of care (SOC).
The FDA encourages the use of ECAs for supplemental approvals in cases where the safety is well characterized through prior clinical trial approvals and for trials with a hypothesis for demonstrating superiority (as opposed to non-inferiority). This is also reflected in the recent FDA guidance regarding Accelerated Approvals within oncology trials that asks for RCTs when investigating new molecular entities. Even in this context, a hybrid design trial with a concurrent control arm would provide the best of both worlds in getting to a successful regulatory approval.
To create an ECA that could viably support a similar regulatory submission, trial sponsors will need to ensure their external patient-level data is clearly defined, well-matched to the applicable research questions, and submitted in an acceptable format. Here are the five steps for building the best possible ECA.
1. Clearly define the scope and objectives of the research questions at hand
Success begins with a concrete and detailed definition of the research questions. What is the exact disease indication under observation? How long will the study observe patients? What is the treatment exposure that qualifies a patient to enter the cohort? What is the clinical outcome and treatment effect estimate that will be measured for these real-world patients?
In a similar vein, researchers will need to consider questions about the role of an ECA at the very beginning of the project planning process, since tacking on an ECA after analysis of the outcomes is frowned upon by regulators to avoid bias in choosing ad-hoc methods for analysis (e.g. cherry picking select patient populations to favor the treatment effect).
Clearly established research questions will help to determine whether an ECA is suitable for a given trial or if a traditional control arm is more likely to produce the desired results.
2. Choose the right ECA design to ensure maximum comparability
Once the team has determined that an ECA is a viable option, it’s time to decide what the cohort should look like. There are several different types of ECAs, including:
- Contemporaneous: Control groups are gathered from data such as registries, EHRs, medical or pharmacy claims, and other RWD sources. These controls may be required for rare disease studies or single arm trials where it is difficult to assemble enough patients to randomize to treatment and/or an active control arm due to practical or ethical reasons.
- Historical: Control groups are generated by pooling data from past RCTs, published medical charts, or other data.
- Hybrid: Control groups are built from a mixture of randomized controls from the clinical trial and external controls from RWD sources. Hybrid ECAs can augment the number of patients assigned to control arms, but they do not fully replace the traditional randomization methodology.
Selecting the right ECA methodology requires careful evaluation within the context of the planned study.
3. Identify fit-for-purpose data sources to architect an ECA
As discussed previously in our blog post “What Makes a Fit-for-Purpose Database for Clinical Oncology Research?”, it is critical to use fit-for-purpose (FFP) data sources throughout the clinical trial.
When using RWD to support an ECA, it is important to note that some clinical elements used for traditional clinical trial criteria are not routinely captured in real-world treatment settings. For example, the Response Evaluation Criteria in Solid Tumors (RECIST) criteria are commonly used in clinical trials, but may not be documented in the same manner in routine community oncology care.
If specific elements are not available, researchers will need to identify proxies or datasets that can be used to supplement existing data to sufficiently address any biases or limitations through methodology, sensitivity analyses, or discussion.
By definition, ECAs involve some degree of data pooling, which may also present issues of quality, completeness, and integrity. RWD vendors should be able to clearly explain how the data was collected and transformed into the delivered dataset.
4. Create an analysis plan
Since selecting an ECA after the completion of a single-arm trial is frowned upon, plan to consult with the appropriate review division as early as possible. The study protocol including the analytic approach should be finalized before initiating the externally controlled trial, with the following design elements should be clearly articulated: the selection of RWD sources, inclusion and exclusion criteria, exposure to control therapy definition, endpoint definitions, and the approaches utilized to minimize missing data and sources of bias.
In the absence of randomization, the investigational and control arm populations need to be as similar as possible based on known patient characteristics that can affect the outcome being measured such as demographic factors, comorbidities, disease severity and prior therapies.
Collection of these baseline and prognostic factors should be complete and comprehensive and similar to the methods employed in the investigational arm of the clinical trial. It is important to maintain statistical rigor in developing a detailed study protocol.
Finally, propose various methods for handling bias and sensitivity analyses to check for the robustness of the assumptions.
5. Understand the detailed requirements for regulatory submission
As the RWD environment evolves, regulatory agencies will carefully scrutinize any submission that includes an ECA. Investigators can get a head start on understanding exactly what the agency is looking for by engaging regulators as early in the process as possible. It is essential to have constructive input from regulators on the study protocol, analytic approach, selection of RWD sources, endpoint definitions, and other key aspects of the project to avoid miscommunication and surprises down the line.
Within the US, this can be achieved by scheduling Type A, B, C meetings or by submitting the protocol for review within the investigational new drug (IND) stage.
During the submission process, researchers will need to show the patient-level data used to create their ECA along with the corresponding data from the investigational arm. The Clinical Data Interchange Standards Consortium’s (CDISC’s) Study Data Tabulation Model (SDTM) is typically used for data submitted to the regulatory agencies, but sponsors may wish to discuss other appropriate data standards and current requirements with the review division, if necessary.
Regulators typically take a very close interest in how the RWD is collected, abstracted, and transformed. Documentation of these processes is expected when submitting a regulatory package, so sponsors should clearly and thoroughly record all their activities from the very start of the project to increase their odds of clearing the high bar of transparency and safety enforced by international regulatory authorities.
How are ECAs being used in the current drug development environment?
ECAs are an exciting use case for RWD, but trial sponsors have only just started to employ this strategy at scale – and only a few regulatory submissions have yet been completed and accepted by global regulators.
ABECMA is one example of how ECAs can potentially inform regulatory decisions. This CAR-T therapy for people with relapsed and refractory multiple myeloma was studied in a single arm trial with overall response rate (ORR) as the primary endpoint. Patient-level data from RWD sources in North America and Europe were pooled together to form an external control arm and compared with the investigational therapy for time to event endpoints of progression free survival and overall survival to establish clinical benefit.
This application led to a successful initial approval with the EMA and was subsequently submitted to the US FDA. The review team noted the substantial heterogeneity of the treatments in the real world setting and considered the data supportive in providing a reference for the expected outcomes of response rates and survival in the real-world setting.
Following these guidelines outlined in creating an ECA and working closely with regulators to meet expectations at every step of the design and execution will help to generate additional evidence for the value of ECAs and further incorporate RWD into the regulatory submissions process.
In our next blog, we will continue the conversation by addressing the different sources of bias to consider when putting together an ECA and how to address them.